The business world is becoming increasingly digitalized, with data analytics and the use of AI now the norm across a wide range of industries.
In order to get ahead and stay afloat in this new and competitive business environment, it’s vital that executives embrace and implement new technologies into their business.
Machine learning, a type of AI that utilizes algorithms to analyze data, is a key technology that is differentiating high-performing companies from their less digitally savvy counterparts.
What makes machine learning unique from other data analyzing programs is its ability to learn from the data, identifying patterns and outliers to improve on its accuracy and make decisions without the need for human intervention.
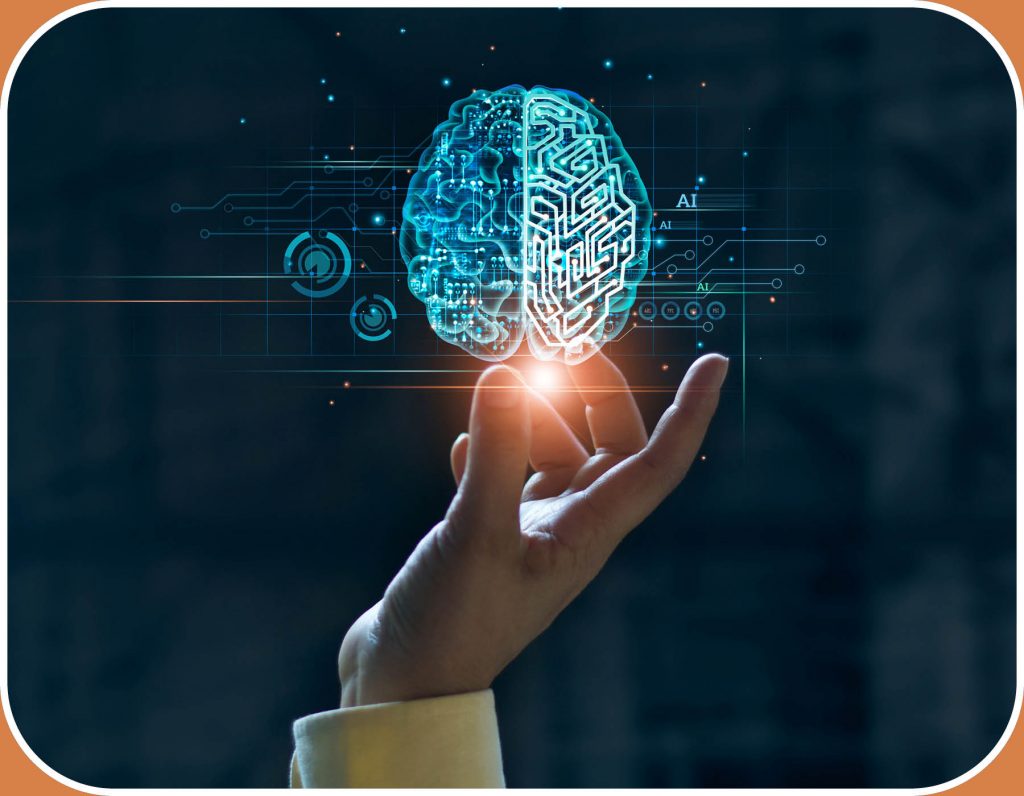
Correctly integrated machine learning can have a multitude of benefits for any business, including that it:
- automates tasks and increases efficiency;
- solves data challenges that impede an organization’s growth;
- improves cyber security;
- achieves personalization without the use of third-party data;
- streamlines the customer experience;
- increases sales and profitability;
- improves risk management.
Here are three founders who have embraced machine learning to create success in their businesses.
Centralizing Data
As Stephany Lapierre watched a client flip through an enormous binder of business cards in search of a key supplier in 2014, it dawned on her how desperately organizations needed access to centralized and transparent supplier data.
“I found it shocking that organizations were spending so much money on suppliers, but very little information was available to them,” she tells The CEO Magazine.
“By the time they discover suppliers, source and run requests for proposals, onboard them, pay them, and manage and maintain their information, we’re talking about millions of dollars being spent each year. And I would say it’s a lot more in lost opportunities or risks.”
Lapierre had the vision to create a single source of truth for supplier data and in 2014, Toronto-based TealBook was born.
“My original thought was to build a platform like LinkedIn that enabled suppliers to put their information in their profile and distribute it to all their customers,” the CEO explains. “But we quickly learned that suppliers wouldn’t do it because we didn’t have the monopoly.”
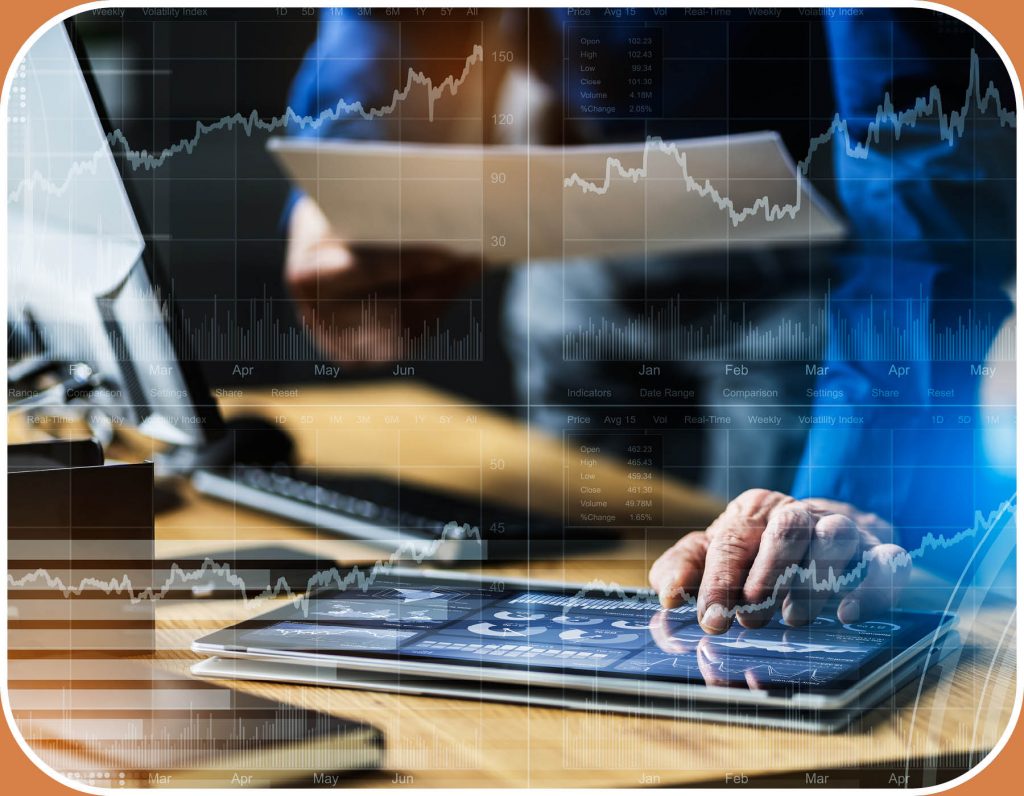
“I found it shocking that organizations were spending so much money on suppliers, but very little information was available to them.”
- Stephany Lapierre
When Lapierre and TealBook’s CTO realized they could build a new platform on Google Cloud Platform and leverage its machine learning services, they knew they’d found the key to unlocking truly dynamic supplier data.
TealBook re-platformed in 2017 after its first seed investment and again in 2021 following a series A funding round. The startup has raised US$73 million to date and saw its revenue grow by 350 percent in 2021 alone.
“Today, we have 5.5 million digital profiles that are continuously being enriched by new data sources we’re adding to include more and more attributes,” Lapierre says.
“We currently have about 73 attributes per supplier at different degrees of quality. The big question at the beginning was: ‘How do we define quality using machines?’
“We can have machines tell us with enough confidence whether an attribute has a high trust score or if it’s too conflicting and our customers shouldn’t depend on it.
“And we’re in the process of adding a lot more transparency, scale and governance so our clients can choose the data they trust and don’t trust. For some clients, high trust at 90 percent accuracy may not be enough. They may need 100 percent.”
Getting The Full Picture
On the other side of the globe, Sydney-based Particular Audience harnesses the power of machine learning to help online retailers create a personalized shopping experience without the use of personally identifiable information.
With third-party cookies set to be phased out, retailers are increasingly looking for effective ways to target consumers without using their personal data.
“Machine learning is effectively predictions, and to make good predictions you need robust data,” Particular Audience Founder and CEO James Taylor explains. “This might seem counterintuitive, but personalization doesn’t require personal data.
“You’re more than your demographic, your location or the time of day you’re browsing. Your context is constantly changing. If you’re buying a Mother’s Day gift for your mother-in-law, your buying behavior will be very different than if you’re shopping for jeans.”
Online retailers already have all the data they need to craft an online journey that shows their customers the products they want to see and boosts their conversions and margins – they just need to find a way to utilize it.
“We can apply a different algorithm to a retailer’s product metadata, product images, and interaction and purchasing data,” Taylor reveals.
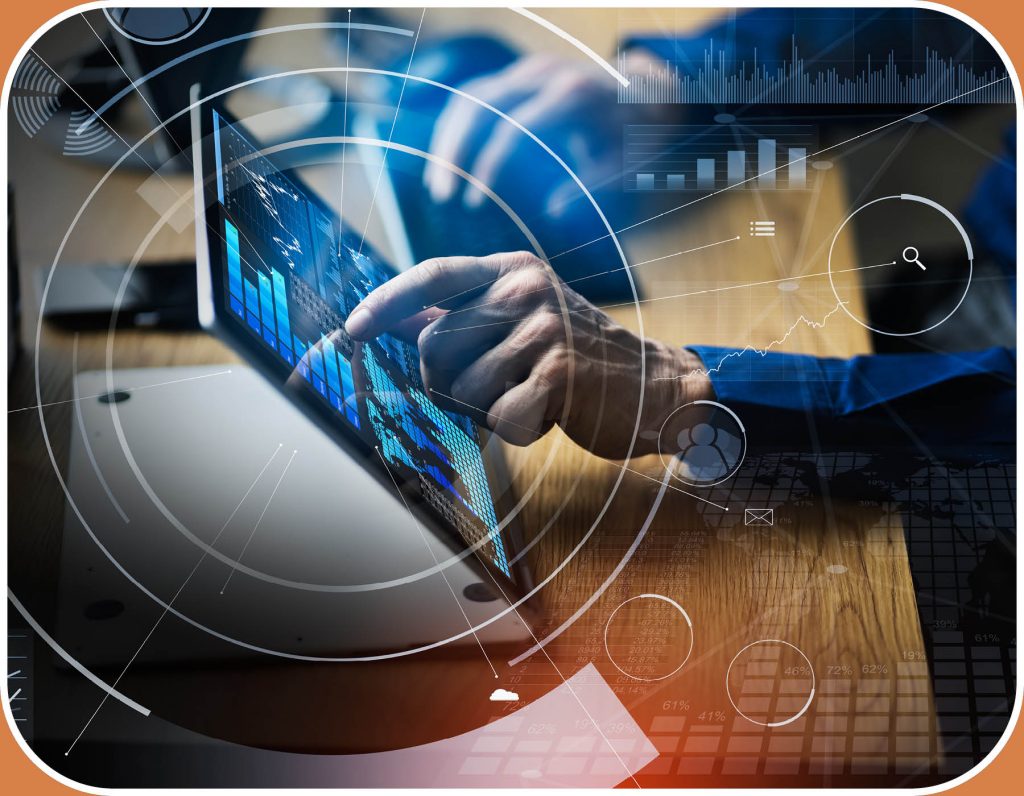
“If you launch ads on your retail website, and that negatively impacts your sales, then you’ve put the cart before the horse.”
- James Taylor
“For product metadata, we use natural language processing to identify items with similar attributes so we can suggest them to the consumer.
“Then there’s computer vision, which is mainly used for fashion and furniture, that allows us to literally go down to the pixel level to understand how visually similar different items are to one another. That’s how you get ‘see similar products’ suggestions.
“Finally, there’s the behavioral data that tells us which items get compared and end up in the same baskets, and allows us to identify comparable, alternative and complementary items.”
Particular Audience sets itself apart from other ecommerce personalization software by allowing multi-brand retailers to charge their suppliers to promote their items within relevant search recommendations.
“Your retail media platform and your search and recommendations personalization shouldn’t be separate,” Taylor points out.
“If you launch ads on your retail website and that negatively impacts your sales, then you’ve put the cart before the horse. Having it embedded into one system is our unique selling point.”
Retailers such as DigiDirect, The Good Guys and Target have seen their units per transaction, average order value and margins soar with Particular Audience’s products. “The results for our Price Beat product are the best we’ve seen,” Taylor says.
“It’s a Google Chrome extension that allows consumers to compare products across different websites and our retailers to undercut the competition in real time. It’s resulted in a 102 percent increase in conversions for products that didn’t already have the best price.”
Reducing Errors
Tiliter is another Sydney-based company that uses computer vision to streamline the supermarket self-checkout process for shoppers and reduce costly errors and fraud for retailers.
“Our AI uses camera images to quickly identify different varieties of produce in grocery stores for shoppers and cashiers,” Co-Founder and CEO Marcel Herz says.
“By increasing the scanning speed, shoppers using our technology can check out five times quicker.
“Another benefit of automatically identifying the item is that customers have much less wiggle room to choose the incorrect item, which might be an accidental or intentional act, which costs retailers millions of dollars a year.
“A popular product in our range is an out-of-the-box AI scale that identifies fruits and vegetables without the need for barcodes or searching through a menu. We also provide AI software that can run on self-service and conventional cashier checkouts.”
With major international supermarket chains including Woolworths, Countdown, Netto and IGA using its game-changing technology, Tiliter has already saved shoppers more than 200 days of waiting in line.
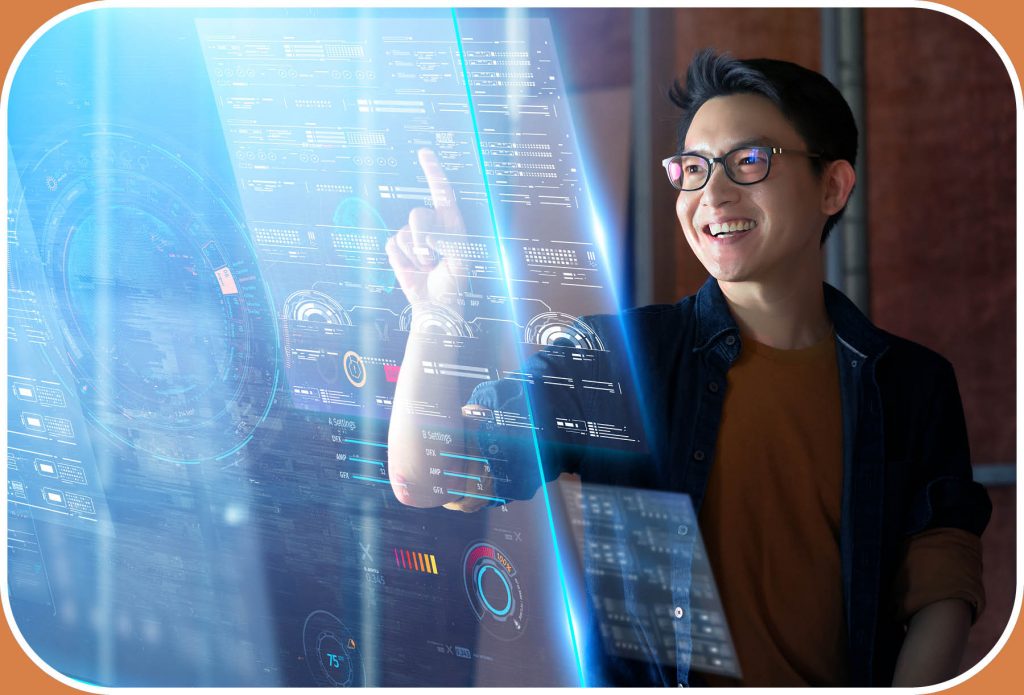
“One day, computer vision will smoothly power the entirety of the supermarket experience.”
- Marcel Herz
“Over the past couple of years, the user experience has become more elegant and user-friendly,” Herz confirms.
“Computer vision was the missing piece for fresh produce, allowing for an even more seamless transaction. One day, computer vision will smoothly power the entirety of the supermarket experience.”
As an increasing number of competitors enter the machine learning market, Tiliter plans to stay ahead of the game through constant innovation.
“Part of our company’s DNA is to innovate,” Herz explains. “Before we started Tiliter in 2017, [CTO] Chris and I studied machine learning applications, one of which was identifying cancer cells in MRI scans via computer vision. It was exhilarating to see the endless opportunities of computer vision.
“Since then, more companies have leveraged machine learning to innovate and improve processes. Fundamentally, this is great for humanity and we want to contribute to bringing its obvious capabilities and usefulness to light.
“Tiliter will continue to innovate, run experiments and provide our customers with better experiences by listening closely to their pain points and holding steadfast in our conviction of where we believe computer vision can take us.”